Gla-AI4BioMed at RRG24: Visual Instruction-tuned Adaptation for Radiology Report Generation
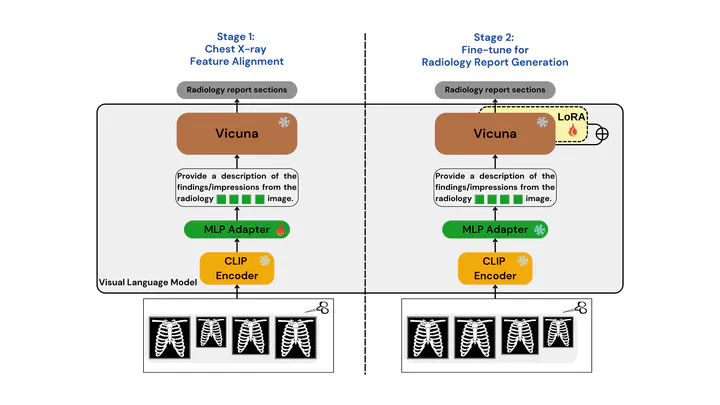
Introduction
Radiology reports are critical tools for interpreting medical imaging, such as chest X-rays. These reports typically consist of two main sections: FINDINGS
, which detail observations from the image, and IMPRESSIONS
, which summarize key takeaways and recommendations.
For example:
FINDINGS:
Increase in size of the left pleural effusion compared to the prior exam. Right lung remains clear. Mildly enlarged but stable heart size.
IMPRESSIONS:
Increase in left pleural effusion. Stable mild cardiomegaly.
Generating such reports automatically is a challenging task that requires aligning visual data with textual descriptions. While general-purpose visual language models like LLaVA and InstructBLIP have shown promise in multimodal tasks, radiology report generation demands a higher level of precision and domain-specific adaptation.
Our approach focuses on fine-tuning a visual language model specifically for radiology. By aligning chest X-ray features with a large language model and applying advanced techniques like Low-Rank Adaptation (LoRA), we enhance the model’s ability to generate accurate and clinically relevant reports. Additionally, we employ a method to process multiple images simultaneously, enabling the model to capture nuanced details across different X-rays.
This work was developed for the RRG24 Shared Task at BioNLP 2024, where our model achieved competitive results, ranking 4th on the leaderboard. Key contributions include:
- Domain-specific fine-tuning: Optimizing the model for radiology tasks through visual instruction tuning.
- Multi-image processing: Combining multiple X-rays into a single input for efficient and accurate interpretation.
Methodology
Our approach builds on insights from LLaVA-Med, emphasizing the advantages of starting with a language-only pretrained LLM rather than a multimodal-trained base. The model integrates an image encoder with a learnable adapter, following the LLaVA-1.5 architecture. Training involves an auto-regressive language modeling approach using cross-entropy loss, with hyperparameters aligned to LLaVA-1.5. We employ Low-Rank Adaptation (LoRA) for efficient fine-tuning, starting with adapter pretraining for one epoch, followed by joint tuning for three epochs.
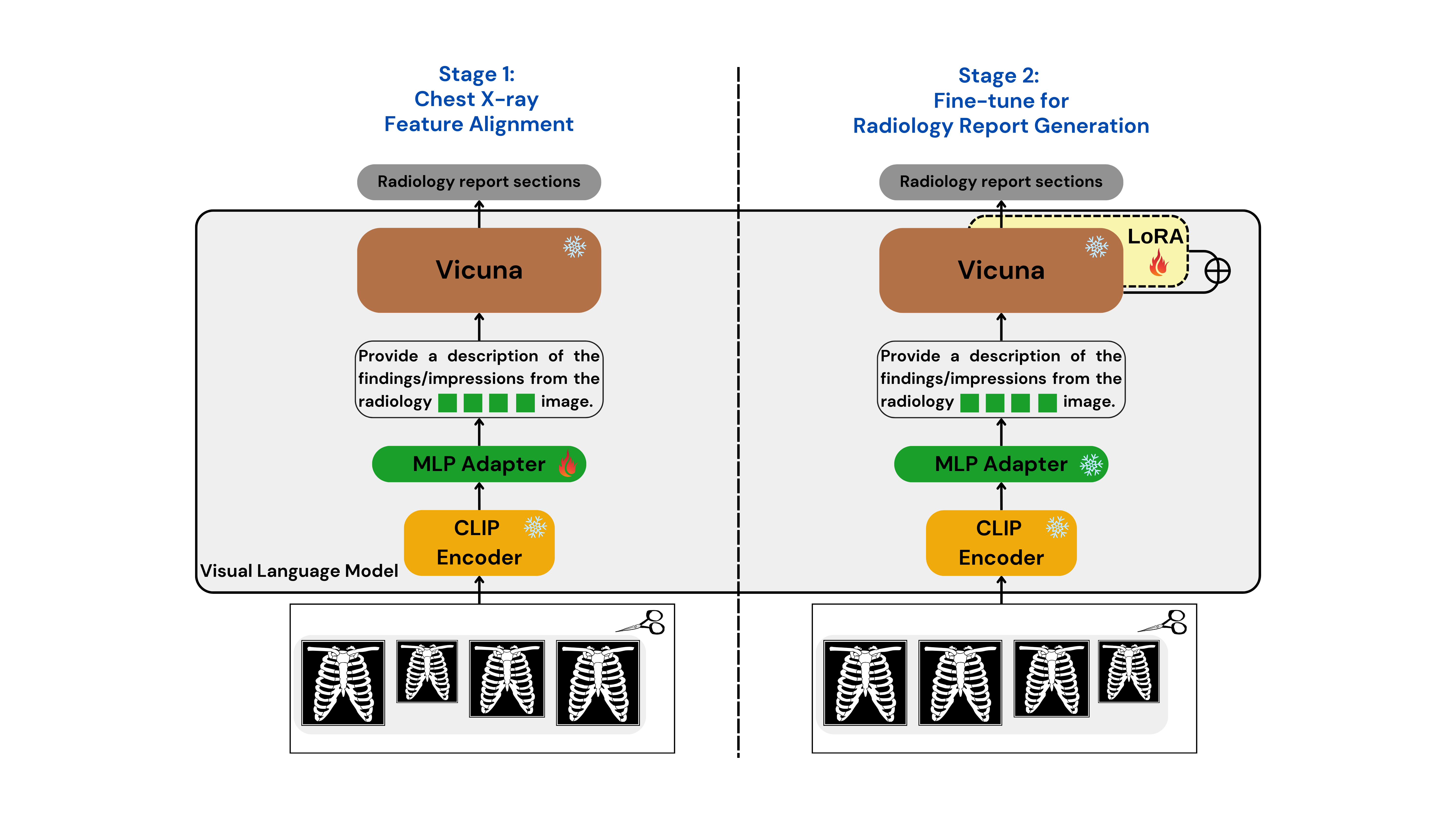
Proposed Models
To tackle the RRG24 shared task, we developed two specialized models: Med-CXRGen-F for Findings and Med-CXRGen-I for Impressions. Both models leverage CLIP as the image encoder and Vicuna-1.5 as the LLM. A multi-layer perceptron (MLP) adapter with GELU activations and a uniform hidden size of 1024 processes image features before aligning them with the LLM.
How It Works
Image Encoding: The image encoder converts chest X-rays into patch tokens, extracting embeddings from the penultimate layer.
Adapter Alignment: The MLP adapter processes these embeddings, aligning them to the LLM’s input format.
Prompt Design: The model is prompted with:
Provide a description of the findings/impressions from the radiology <image>\n image.
Here, "<image>" acts as a placeholder token, guiding the LLM to generate text based on the input image, as shown in Figure.
Training
Our training process involves a two-stage approach to optimize the model for radiology report generation: (as shown in Figure)
Chest X-ray Feature Alignment
In the first stage, we align chest X-ray image features with textual embeddings in the language model. Using the provided dataset, the model predicts captions based on image inputs and instructions. During this phase, only the MLP adapter is updated, while the visual encoder and LLM weights remain frozen. This single-epoch training expands the vocabulary of aligned image-text tokens specific to the radiology domain.Fine-tuning for Report Generation
In the second stage, we fine-tune the pre-trained LLM weights using LoRA technology. The visual encoder and adapter weights are kept frozen, while the model undergoes three epochs of training on the dataset. This phase focuses on enhancing the model’s ability to generate accurate and clinically relevant radiology reports.
Evaluation
Dataset
Our models were fine-tuned and evaluated using the RRG24 dataset, hosted on the BioNLP ACL'24 platform. This dataset aggregates data from multiple sources, including MIMIC-CXR, CheXpert, PadChest, BIMCV-COVID19, and OpenI. The dataset statistics are summarized below:
Dataset | FINDINGS | IMPRESSIONS |
---|---|---|
Training | 344,394 | 366,413 |
Validation | 8,839 | 9,331 |
Test-Public | 2,692 | 2,967 |
Test-Hidden | 1,063 | 1,428 |
Results
Our models achieved competitive F1-RadGraph scores of 24.13 and 22.79 for the Findings and Impressions sections, respectively, on the public test set. On the hidden test set, the scores were 24.13 and 22.10, securing 4th place on the leaderboard at the time of submission. Additionally, the Bertscore results highlight the high-quality text generation capabilities of our models, with scores of 53.45 for Findings and 47.39 for Impressions on the public test set.
Discussion
The difference in performance between the Findings and Impressions sections comes down to their unique roles. Findings are all about objectively describing what’s seen in the images, while Impressions focus on drawing diagnostic conclusions. This difference, along with the varying lengths of these sections, makes generating Impressions a bit trickier, which is reflected in the evaluation scores.
Another factor is the variety of diseases in the test set. This uneven distribution can make it harder for the model to generalize well. Plus, when the model processes multiple images at once, it sometimes includes irrelevant ones, which can throw off its accuracy. Improving the model’s ability to filter out unnecessary images could make a big difference.
Looking ahead, there’s a lot of room for improvement. Fine-tuning the model specifically for medical data and adapting it to better handle domain-specific challenges could help. Adding the ability to track changes over time and creating smarter frameworks for multi-modal reports are also exciting directions to explore. These upgrades could make the model even more useful and reliable in real-world clinical settings.
Conclusion
In this study, we introduced a model designed to generate radiology reports by aligning visual and textual data. By fine-tuning it for specific tasks, we managed to achieve solid results, including a fourth-place finish in the RRG24 Shared Task at BioNLP 2024. This shows the potential of our approach for specialized medical applications.
Moving forward, we’re excited to explore ways to make the model even better. This includes developing methods to handle multi-modal data more effectively and incorporating time-based insights. These improvements could take the model’s accuracy and practicality to the next level for clinical use.
Limitations
While the results are promising, there are a few challenges we need to address:
- Easier-to-Detect Conditions: Some diseases are simpler to identify, which might inflate the evaluation scores.
- Dataset Imbalance: The training data isn’t perfectly balanced in terms of imaging types, body parts, or report lengths, which could impact performance.
- Inconsistent Reporting Styles: Radiologists have different ways of writing reports, and this variability makes it harder for the model to generate consistent outputs.
BibTeX
@inproceedings{zhang-etal-2024-gla,
title = "Gla-{AI}4{B}io{M}ed at {RRG}24: Visual Instruction-tuned Adaptation for Radiology Report Generation",
author = "Zhang, Xi and
Meng, Zaiqiao and
Lever, Jake and
Ho, Edmond S.L.",
editor = "Demner-Fushman, Dina and
Ananiadou, Sophia and
Miwa, Makoto and
Roberts, Kirk and
Tsujii, Junichi",
booktitle = "Proceedings of the 23rd Workshop on Biomedical Natural Language Processing",
month = aug,
year = "2024",
address = "Bangkok, Thailand",
publisher = "Association for Computational Linguistics",
url = "https://aclanthology.org/2024.bionlp-1.54/",
doi = "10.18653/v1/2024.bionlp-1.54",
pages = "624--634",
}